next on phyloseminar.org
To attend a seminar, please visit our YouTube channel.
Ancestral recombination graphs 2
Scalable approaches to inference and analysis of genome-wide genealogies
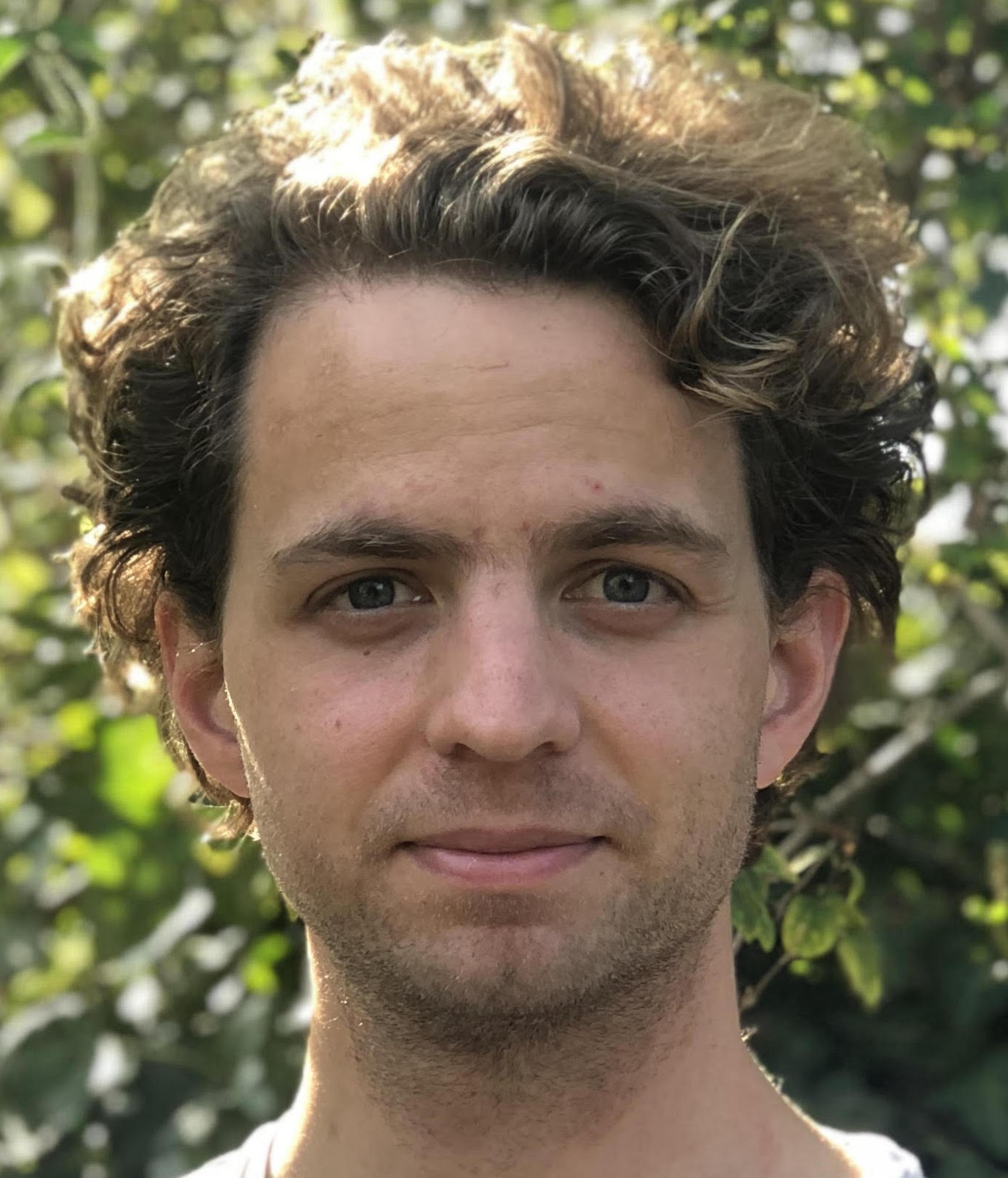
The ancestral recombination graph (ARG) is a graph-like structure that encodes a detailed genealogical history of a set of individuals along the genome. ARGs that are accurately reconstructed from genomic data sets are useful for a range of applications in statistical and population genetics, but inference from data sets comprising millions of samples and variants remains computationally challenging. In this talk, I will introduce a novel ARG inference algorithm, called Threads, and show how ARG inference can be applied to bionbank-scale data sets using the algorithmic paradigm of “threading”. Using inferred ARGs, I will then explore applications of inferred ARGs to three familiar tasks in statistical genetics. First, I will show how threading algorithms can be used to improve upon traditional genotype compression methods by identifying long identical-by-descent segments. Second, I will show how careful modeling of allele ages can help improve imputation of ultra-rare variants. Finally, I will discuss how inferred ARGs can complement or improve upon traditional genetic association studies.
Bayesian inference of Ancestral Recombination Graphs: progress and challenges
Ancestral Recombination Graphs (ARG), or sometimes known as Genome-wide Genealogies, describe the full genealogical history of the genomes and are richly informative about the evolutionary history. Recent years we have witnessed great progress in scalable inference of ARG on thousands or more genomes. However, many of them lack accuracy and can be sensitive to model mis-specification from demographic histories or selection. Moreover, they reconstruct only a single ARG topology and cannot quantify the considerable estimation uncertainty in ARG inference. To address these challenges, we introduce SINGER, a novel method which accelerates posterior sampling of ARG by highly optimized MCMC for at least hundreds of genomes. In this talk I will demonstrate the enhanced accuracy and robustness to model mis-specification of SINGER, and give examples of applications to real data. These examples include various aspects of evolutionary biology, such as demography, positive selection, balancing selection, and introgression, etc. Last but not least, I will discuss possible directions of pushing Bayesian inference of ARGs even further.
Untangling ancestral recombination graphs to infer complex demographic scenarios
The ancestral recombination graph (ARG) encodes the complete genealogical relationships among a set of recombinant DNA sequences; and is therefore a (very high-dimensional) sufficient statistic for many evolutionary and population genetic models. Over the past decade, numerous methods have been developed to infer an ARG (or partial ARG) from phased variants, often using heuristics for the sake of scalability. There are two major obstacles to the use of these 'empirical' ARGs for subsequent model-based inference (for example, to fit and evaluate complex demographic models). First, error in ARG reconstruction is inevitable and difficult to characterize; second, the likelihood of a parameterized model given an ARG is challenging to compute even in the absence of error. I describe a statistical framework which mitigates these issues by viewing ARGs as collections of dated events (e.g. coalescences) from which simpler time-to-event distributions can be readily approximated. Heavily parameterized models may be fit by matching empirical and expected hazard functions of marginal time-to-event distributions, using standard methods from the theory of continuous-time Markov processes. More generally, this approach projects an ARG to a set of time-indexed summary statistics that are highly informative while remaining robust to reconstruction error.